Generative AI and LLMs in Banking: Examples, Use Cases, Limitations, and Solutions
.webp)
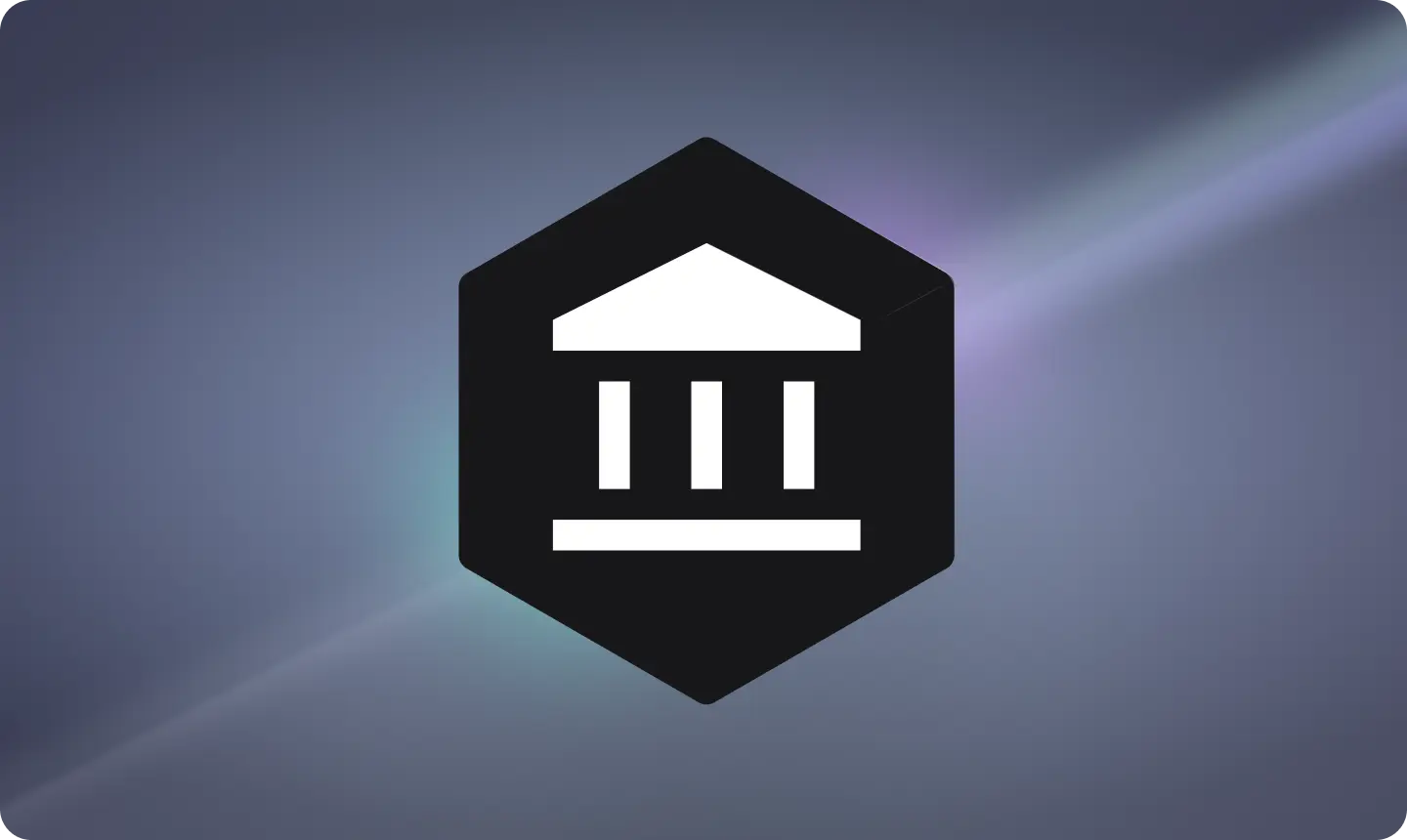
Banking may not seem like the most technologically progressive industry. But it isn’t slow; it’s just very cautious. If we look at the tech evolution of financial institutions, we’ll see they are riding the tech wave—with a life jacket on. We’ve witnessed this slow adoption of new developments with online banking, cloud services, and paying with an Apple Watch.
Artificial intelligence is the latest technology to keep the financial industry on its toes. But riding the wave doesn’t mean leaping in headfirst. So, while some big banks—like Wells Fargo, Capital One, and JPMorgan Chase—have tested the waters with GenAI, many others are still hesitant.
We’re not here to aggressively push bank executives into the uncharted waters of GenAI or, more specifically, large language models (LLMs)—that’s not our style. What we want to do is give you the information to make an informed decision. So stay tuned to learn more details about the use cases of generative AI in finance, the limitations that may hinder its adoption, and the banks that have embraced LLMs and love them.
The State of GenAI in Banking
Most banking institutions are currently figuring out the best ways to use generative AI and LLMs effectively and ensure they get adopted within their organizations.
A recent report by The Alan Turing Institute shows that the UK financial sector has been reluctant to adopt LLMs. The workshop this research was based on consulted 43 banking industry professionals and uncovered the following:
- Slightly over 50% of them apply LLMs to enhance performance in information-focused tasks
- 29% employ LLMs to bolster their critical thinking abilities
- 16% utilize LLMs to simplify complex tasks
- 10% leverage LLMs to improve collaboration within their teams
- 35% do not use LLMs
Modern LLM use in the UK financial sector mainly involves risk-free tasks with human assistance, such as text summarization and analysis acceleration. While some firms use LLMs for internal purposes like training, they are not widely used for direct customer service provision yet.
However, the sector is on the verge of significant change, with the integration of LLMs expected across all functional areas within five years (most workshop participants anticipate this integration happening within two years). KPMG reinforces this opinion, stating that 60% of financial executives expect their first GenAI solution to be implemented in 1-2 years.
The experts at McKinsey also predict an important impact of GenAI on the banking industry: an additional $200 to $340 billion per year in revenue, mainly due to improved productivity.

At the same time, the market for finance-focused generative AI applications is already growing. Research suggests that the global market size for GenAI in finance will rise from $1.19 billion to $13.33 billion in the next ten years. So, it’s not surprising that industry veterans with decades of experience and terabytes of data are already working on artificial intelligence models for banks.
Take Bloomberg’s large-scale GenAI model designed specifically for the financial sector—BloombergGPT. The model has over 50 billion parameters and has been trained on a comprehensive data set of over 700 billion tokens, making it one of the largest domain-specific language models available.
It was developed to help financial institutions with various natural language processing tasks (NLP) like sentiment analysis, named entity recognition, news classification, and question answering.
All this leads us to consider how banks can use generative AI with the highest risk-to-benefit ratio.
Possible Use Cases of GenAI and LLMs in Banking
GenAI has thousands of potential applications in the banking sector. We didn’t say it—KPMG did. Their report on the benefits of GenAI in the financial services sector found that 76% of banking executives in the US plan to use generative AI for fraud detection and prevention, 62% for customer service, and 68% for regulatory compliance and risk avoidance.
KPMG also discovered that GenAI shows better results than traditional AI models when it comes to:
- Content summarization
- Conversational knowledge
- Content generation
- Code creation
- Sentiment analysis
- Natural language interface
- Deep learning
- Digital twins
Overall, we see the following leading use cases for generative AI and LLMs in banking.
Enhanced Customer Experience
LLMs can help improve the online banking experience through efficient and empathetic service. Using advanced natural language processing, LLMs can analyze a client’s account, understand their intent, and offer fine tuned solutions in real time, increasing satisfaction and loyalty.
Banks can use LLM-powered chatbots for 24/7 personalized support, text generation, financial advice, and routine inquiry management to free up human agents, allowing them to focus on complex or specific requests. And thanks to generative AI assistants, the support team can quickly access relevant account information, product guides, or policies to address client queries more quickly.
Example: Klarna
Klarna, a Swedish BNPL company, boasts an AI-based customer service assistant integrated into their app. The OpenAI-powered assistant handles various requests and tasks, from helping clients select products to managing refunds and disputes. Thanks to generative AI models, the assistant understands and responds to customer queries in a way that resembles human interaction.
The assistant is scalable and accessible. It can hold conversations in 35 languages and operates around the clock to provide continuous support to old and new customers, regardless of their time zone or geographical location.
Back Office Automation
GenAI-based virtual assistants can help bank employees find and make sense of complex information in contracts and other documents, like policies, credit memos, and trading information. Plus, it helps automate data extraction from documents such as loan applications and financial statements, reducing errors and speeding up their processing.
GenAI can also retrieve information from internal databases or the Internet, write code, summarize long documents, and generate templates so employees can make informed decisions or create reports faster. And don’t forget about its unparalleled skill in generating content for all kinds of marketing and social media activities.
Example: SouthState Bank
Florida’s SouthState Bank has trained ChatGPT on its data and internal documents to help employees quickly access and analyze internal information. The bank’s employees use the tool for tasks such as composing emails, creating expense reports, detecting suspicious activity, and analyzing potential fraud. It also helps to summarize policies, understand regulatory documents, and create marketing materials.
Overall, the use of ChatGPT has reduced task completion times from 12-15 minutes to just seconds and improved the bank's productivity by 20%.
Financial Analysis and Risk Management
Using LLMs, banks can analyze extensive volumes of unstructured data, including news articles, reports, internal documents, and regulatory filings, to forecast trends and assess market risks. This improves risk management, reduces exposure to market volatility, and augments regulatory compliance, resulting in higher returns and better financial performance.
Example: Deutsche Bank
Deutsche Bank has recognized the potential of generative AI and is using it to improve various areas of its business processes. In particular, the bank focuses on improving risk calculations using GenAI's fast data processing and analysis capabilities. In addition, the bank is developing software that improves developer productivity and using AI chatbots to handle employee and customer queries based on text and voice data. These initiatives provide insights into customer behavior, streamline internal workflows, and strengthen the bank’s risk assessment processes.
Detecting suspicious behavior and fraud
Generative AI assists banks in detecting fraud by analyzing data to identify unusual patterns indicative of suspicious activity. They monitor transactions and customer communications in real time, allowing banks to quickly investigate anomalies that may signal scams or account manipulation. By recognizing known fraud patterns and adapting to emerging threats, these models effectively reduce false positives and protect customer accounts and assets.
Example: Mastercard
Mastercard uses GenAI to improve its fraud prevention Decision Intelligence system, which currently processes 143 billion transactions per year.
The upgraded system scans up to one trillion data points and analyzes the relationships between entities within transactions to determine potential risks more accurately. This new technology provides an improved assessment of account, merchant, and device data, increasing fraud detection rates by up to 20% on average and, in some cases, by as much as 300%.
Faster Loan Application and Creditworthiness Assessment
LLMs can speed up banks' creditworthiness assessments by analyzing a wide range of complex information, including money transaction history, spending patterns, and social data. This enables a nuanced evaluation that gives lenders a clearer picture of risk while ensuring that borrowers with limited credit histories are considered more fairly.
Another use case for banking-specific LLMs is helping people fill out loan or mortgage forms. GenAI-based chatbots can guide borrowers through perplexing processes while bank workers can enjoy verified information and automated mortgage applications. The models dynamically adapt to new data, streamlining the lending process and reducing costs, leading to more comprehensive and accurate lending decisions.
Example: Westpac
Australia’s Westpac is working with Kasisto to test KAI-GPT, a bank-specific LLM designed to facilitate customer support and internal operations. Westpac will deploy KAI-GPT internally, primarily to support bankers, customer support agents, and mortgage officers. Initially, the AI will assist with mortgage applications, clarifying forms, and required information to speed up the lending process.
KAI-GPT will also reduce errors by refining answers using industry-wide data and Westpac's proprietary information. This layered training approach ensures the system can draw on a broad range of banking knowledge while providing specific guidance based on Westpac's forms, policies, and documents.
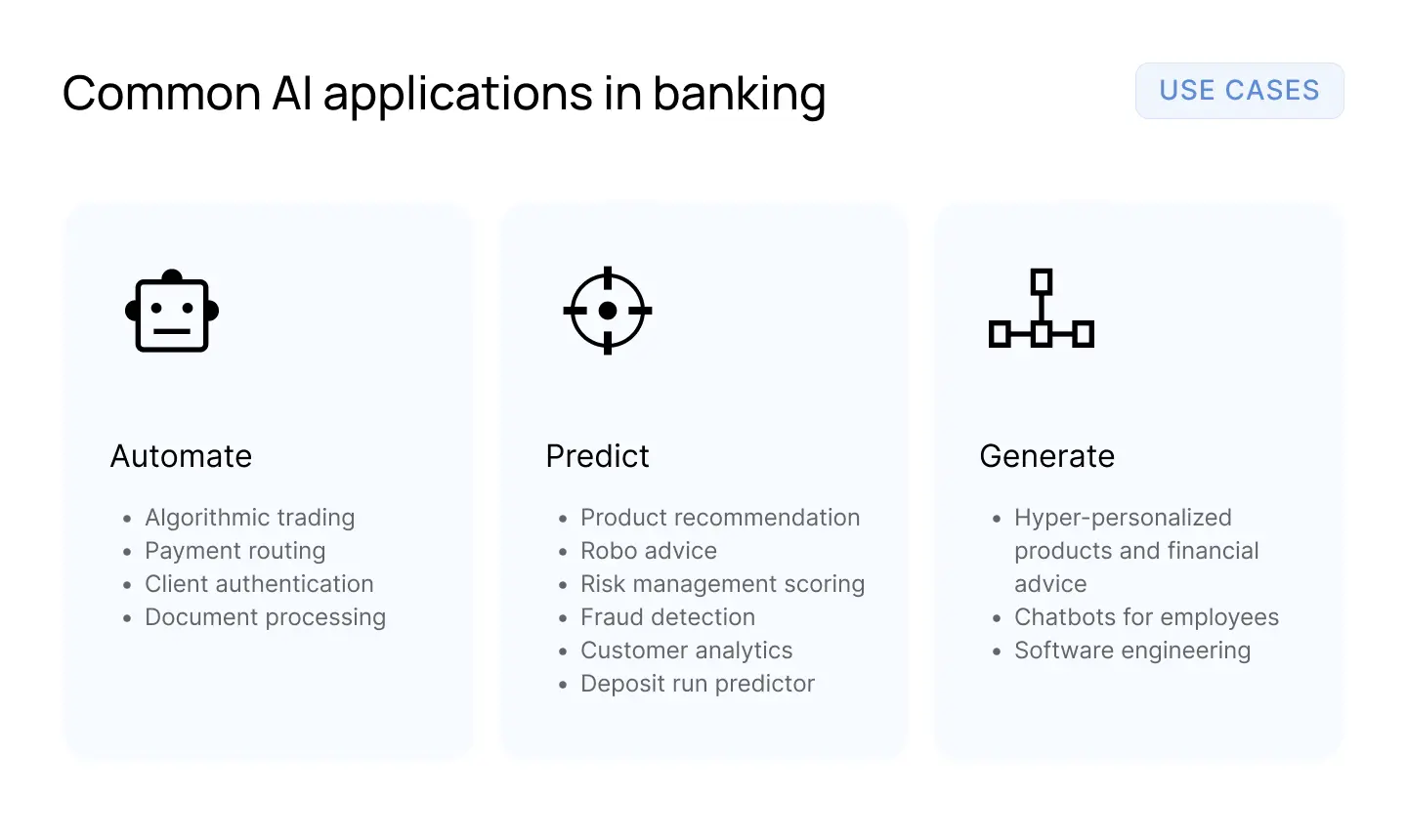
The ROI of LLMs and GenAI in Banking
KPMG reports that within the next year, 97% of banking leaders are gearing up to invest in GenAI. Nearly a quarter of them plan to allocate $100-249 million, while 15% are eyeing investments between $250-$499 million. Furthermore, 6% are looking to invest over $500 million.
The primary areas of investment focus include:
- Improving customer experience (45%)
- Training and developing the workforce (35%)
- Acquiring GenAI technology and solutions (30%)
- Establishing responsible GenAI governance programs (30%)
In 2022, the KPMG US Technology Survey found that the banking industry expects an ROI of more than 50% from investments in AI tech. And their 2023 Global Tech Report suggests that banking tech leaders already see the positive effects of artificial intelligence and automation on profitability and performance.
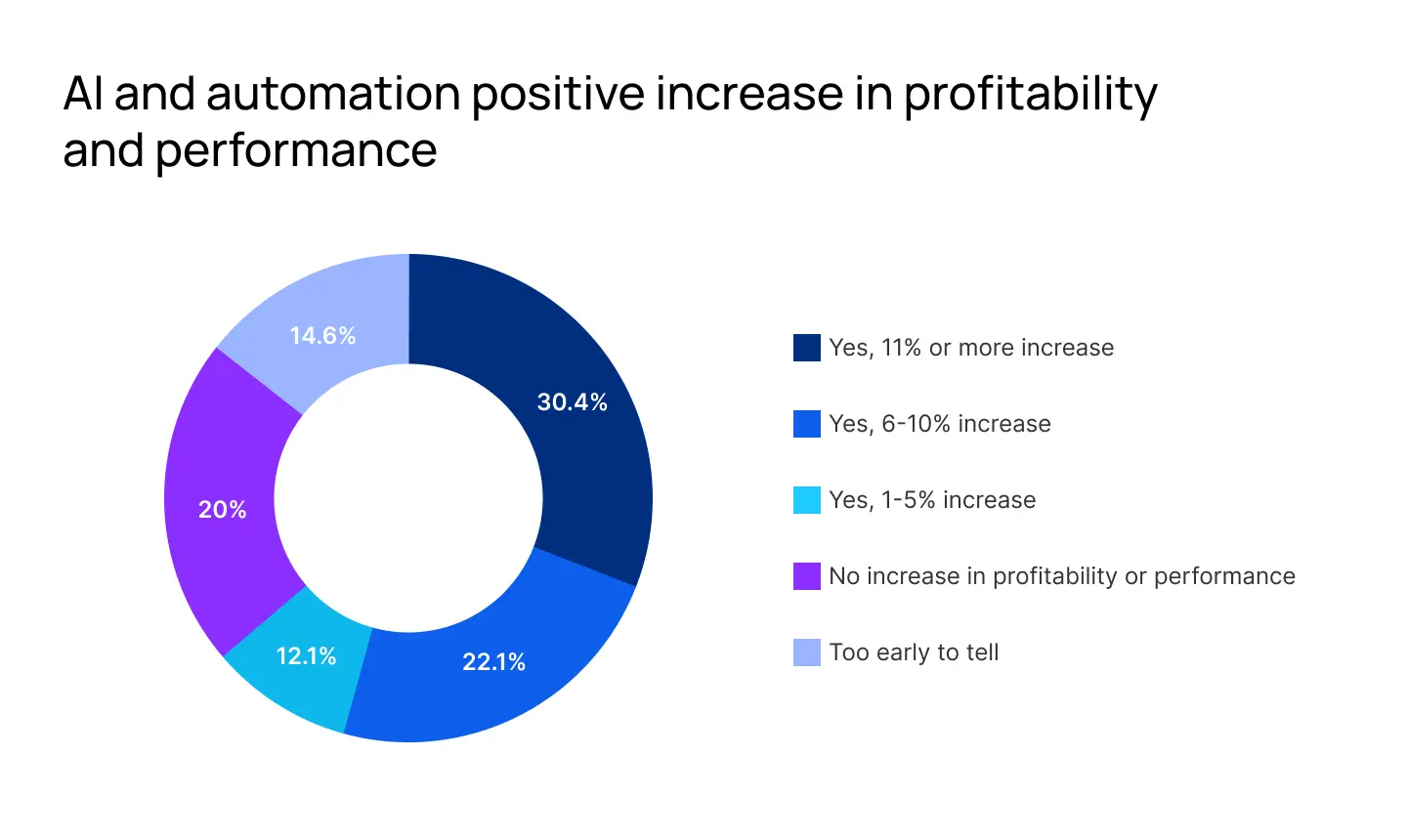
Half of the surveyed leaders anticipate that the most significant value generated from GenAI investments will come from analyzing customer data to improve current products and services. Other areas expected to yield value include:
- Boosting efficiency for increased productivity (48%)
- Enhancing product quality, efficiency, and innovation (42%)
- Streamlining supply chain operations to cut costs (37%)
Currently, half of the surveyed banking managers measure the return on their investment in GenAI in terms of increased productivity (51%), with employee satisfaction (48%) and revenue growth (47%) following closely.
Moreover, there is anticipation among 90% of leaders that GenAI could help alleviate employee stress and burnout. Indeed, improved employee satisfaction is the main benefit of AI and automation among the surveyed bank technology leaders.
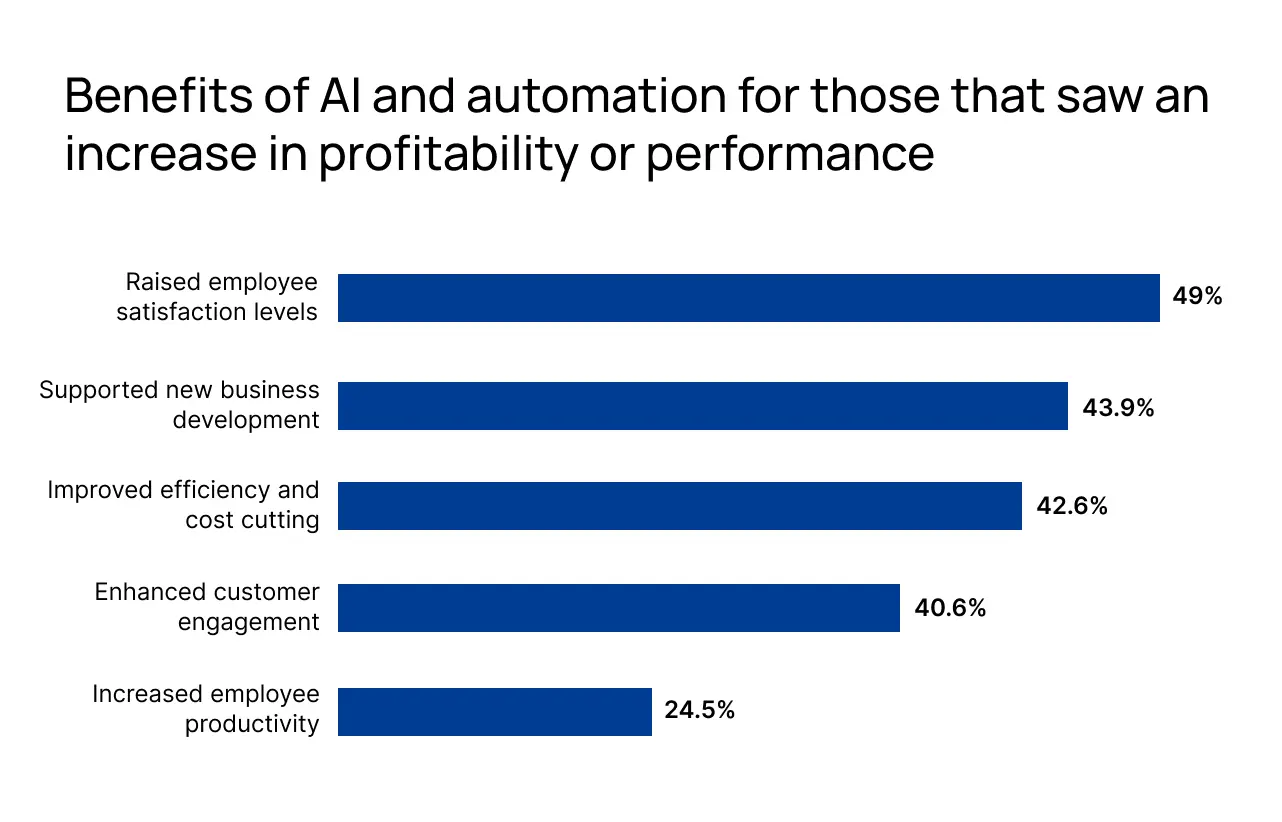
Limitations to GenAI Adoption in Banking
The successful use of generative AI in financial technology requires a careful balance between innovation and mitigating challenges. Here are the difficulties banks cite most often:
- Bias and fairness issues: GenAI models trained on vast data sets can perpetuate biases, leading to unfair customer treatment, especially in credit scoring or loan approvals. However, this issue can be mitigated with careful data selection and transparency.
- Interpretability and explainability: The black-box nature of many large language models complicates regulatory compliance and risk management, making their decisions challenging to interpret.
- Resource intensiveness: Implementing LLMs demands high computational resources, often unaffordable for smaller banks.
- Reliability. Generative AI can answer the same prompts differently, often without citing sources. This makes it hard to believe the output is accurate, as we’ve all heard how GenAI can hallucinate.
While addressing all these pitfalls is important, security concerns plague GenAI adoption in banking the most.
GenAI Security Concerns…
It is easy to damage a reputation, and reputation is crucial for banks. If word gets out that a bank has security problems, it will be very difficult to win back the trust of customers. This is the main reason banks are not rushing to exploit the full potential of LLMs.
Sharing sensitive data with providers such as OpenAI poses significant risks for banks due to several factors:
- Data privacy and security: Generative AI models require extensive training data, but the information banks process is very sensitive. When such information is shared with third-party providers, there is a risk of unauthorized access or data breaches, which is why robust encryption, access controls, and anonymization are essential.
- Cybersecurity threats: LLMs are prime targets for cybercriminals who exploit vulnerabilities to manipulate data. While generative AI can improve fraud detection, it also poses new cybersecurity risks. CEOs fear the technology is a double-edged sword that improves defenses but also opens up new vulnerabilities.
- Third-party risks: Banks often depend on third-party vendors and digital tools for AI infrastructure, which introduces additional risks such as supply chain attacks and vendor vulnerabilities.
Of course, banks must rigorously vet their AI partnerships, strengthen security measures, and ensure compliance to minimize risks associated with sharing sensitive data. But there’s a more effective solution to GenAI-related security concerns.
…And Solutions
Hosting large language models on-premises addresses data security issues and could encourage banks to use GenAI for more than just customer chatbots. By storing sensitive customer data within their infrastructure, banks strengthen their data privacy and security while also complying with regulatory requirements governing data protection.
Training LLMs on premises allows banks to optimize model performance and accuracy and tailor models to their needs thanks to proprietary data, domain-specific knowledge, and internal expertise. Additionally, reduced reliance on third-party vendors minimizes risks like vendor lock-in, service interruptions, and limited control over data processing.
Not to mention, banks that guarantee data privacy and employ ethical AI practices demonstrate reliability, integrity, and accountability. This also promotes transparency and trust among stakeholders, including customers, regulators, and internal decision-makers.
Despite the upfront investment required, on-premises hosting offers scalability and long-term cost savings, enabling banks to develop customized AI solutions without the risks of data sharing for their business.
Example: BNP Paribas with Mistral AI
French generative AI startup Mistral AI raised €385 million in funding, with significant participation from BNP Paribas. Mistral AI boasts a transparent, open-weights model that clients can deploy on their own infrastructure, ensuring sensitive data remains secure within their premises.
Conclusion
KPMG says that generative AI is still in its PoC stage, and we agree. Though we have yet to see what LLMs are fully capable of, everything points to the fact that banks consider it a solid investment and will keep experimenting to adapt GenAI to their business workflows. However, we always advise banks to use custom on-premises LLMs to safeguard sensitive data effectively.
Dynamiq simplifies the challenges of deploying and managing large language models on-premises. By empowering banks to develop GenAI applications on their own infrastructure, Dynamiq allows them to maintain complete data control and comply with regulatory requirements. We help financial institutions make data-driven decisions by providing easy access to information, efficient compliance management, and customizable workflows. So, if that’s something your bank is interested in, contact Dynamiq. We have a lot to offer.