Balancing Innovation and Privacy: LLMs under GDPR
.webp)
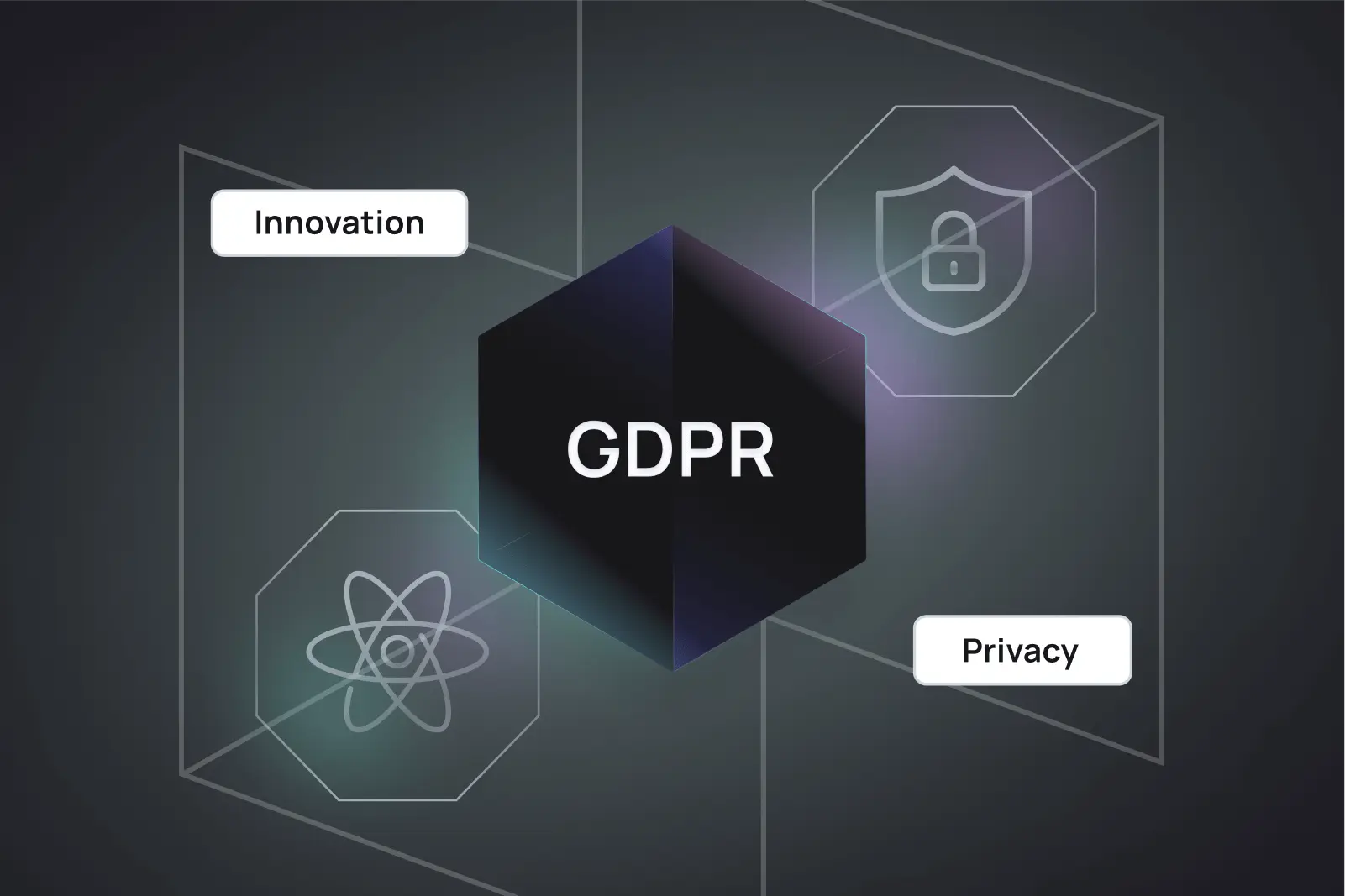
When a new technology emerges, the technical team is usually more enthusiastic about implementing it than the C-suite. But in the case of generative AI, the enthusiasm actually comes from the top down: This time, it’s the business leaders and stakeholders who want to implement AI, and chief security officers or heads of data security are on the fence. And it’s easy to understand why.
When a company implements generative AI, especially one operating in or targeting the European Union, it must have a thorough understanding of the General Data Protection Regulation (GDPR). Otherwise, it may face the notorious GDPR fines.
What are the challenges for LLMs under the data protection law, and what are the solutions? Let’s find out together.
Productivity Boost and Insights: How Enterprises Use LLMs
The integration of large language models (LLMs) in enterprise environments is growing slowly but steadily. Ernst & Young reported that of businesses investing in AI, 80% are developing proofs of concept (POCs) for various applications, and 20% are engaged in pilot projects.
Enterprises in industries like finance, healthcare, retail, and marketing use generative AI and LLMs for:
- Automating customer service. LLMs can understand routine customer queries, manage requests, and provide support, freeing human employees to handle more complex problems.
- Creating content. From generating reports to drafting emails to creating marketing content, LLMs save time and money.
- Analyzing data and attaining insights. LLMs can process and analyze large amounts of data to predict trends, reveal insights, and support decision-making.
- Improving research. By summarizing large amounts of text, LLMs help with research and development work.
- Streamlining processes. LLMs can automate routine tasks, manage workflows, and optimize logistics, contributing to smoother operations.
However, the widespread corporate adoption of generative AI functions is progressing more slowly than expected. MIT Technology Review Insights and Telstra’s global survey of over 300 business leaders found that among international organizations, only 9% are using AI to any significant extent. According to 77% of respondents, regulatory, compliance, and data privacy environments are key barriers to the rapid adoption of generative AI.
Data Privacy Concerns
Integrating LLMs in business processes brings challenges in terms of personal data management and data protection principles. The challenges include:
- Volume and variety of data. LLMs require vast amounts of data for training and efficient operation. This often includes personal data, which ranges from basic identity information to more sensitive data, such as individual preferences, behavior, and biometrics.
- Opacity of AI models. LLMs, especially those based on deep learning, can be "black boxes" with automated decision-making processes.
- Persistent and evolving threats. Unauthorized access and data breach incidents are major cybersecurity risks, among others, associated with big data storage and processing.
How does GDPR factor in? While General Data Protection Regulation may intimidate some businesses working with the data of EU citizens, it simply determines how companies should implement GenAI in a legally compliant manner.
GDPR Considerations for Implementing LLMs
When using LLMs, enterprises must abide by GDPR limitations on the use of private data. How can they manage the complex intersection of data subjects, technological innovation, and regulatory compliance? Let’s see.
Privacy and Data Protection Compliance
According to GDPR, the use of personal information must be lawful, fair, and transparent, requiring explicit consent and holding a defined purpose with stringent security measures.
Businesses have a legal obligation to obtain consent for data use, ensure the accuracy of the data, and protect confidentiality. Designers of AI systems must ensure privacy by using measures like encryption, secure storage, and controlled access.
Personal Data Rights
GDPR offers people control over their personal data. For example, it allows them to access and correct their information or request its deletion. However, the nature of LLMs makes it difficult, or impossible, to track and remove specific data points. Thus, companies need systems that allow them to trace the origin of the data used to train their models and handle any requests from individuals to update or delete their data.
Risk Assessment
GDPR requires Data Protection Impact Assessments (DPIAs) for activities that could significantly risk people’s privacy and rights. Therefore, companies must assess and mitigate the risks before processing personal data. This is particularly important when working with sensitive data or new technologies.
Transparency and Accountability
The General Data Protection Regulation requires transparency about how enterprises use LLMs and their role in decision-making processes, especially regarding personal data and individuals' rights. Therefore, organizations need to clearly communicate AI's role and capabilities in data collection and obtain explicit consent for the use of personal data.
Data Minimization and Purpose Limitations
GDPR stresses the importance of collecting personal data only for the necessary, specific, clearly defined purposes. So, enterprises must clearly communicate the purpose of data collection and use, configure LLMs to prevent excessive data collection, and ensure data is used only for its stated purpose.
And here’s a tip: whenever possible, companies should use anonymized or synthetic data, which is not considered personal data under GDPR.
Security Measures
GDPR requires strong security measures to protect personal data, including data used by LLMs, from unauthorized access and breaches. Common techniques include encryption, anonymization, and pseudonymization. Companies should train their employees on GDPR requirements, especially employees working with AI systems, to ensure compliance and prevent breaches.
As you can see, GDPR plays a crucial role in shaping how companies operating in any EU member state approach the use of LLMs. Instead of prohibiting the use of GenAI, GDPR only provides guidelines for its implementation. These guidelines do come with some challenges, though.
GDPR Challenges for LLMs
Understanding the challenges of GDPR compliance in LLM implementation requires a detailed examination of the data lifecycle associated with training and deploying these models. Specific areas of concern include training data, inferences from prompt data, and inferences from user-provided files.
Training Data
Training data is foundational for developing effective LLMs. And because training data can include vast amounts of personal information collected from various sources, organizations must establish a lawful basis and method for processing it. Where possible, privacy risks should be reduced by anonymizing or pseudonymizing the data without allowing it to be re-identified.
Inference from Prompt Data
When LLMs generate output from user prompts, they process and may store any data provided in the prompt, which can include personal information. So, it is critical to ensure the security of such prompt data to prevent breaches.
GDPR mandates that personal data be kept for no longer than necessary. This means organizations must define and enforce minimum retention policies. In addition, the use of data provided in prompts must be strictly limited to the purpose that is explicitly stated and to which the user consents.
Inference from User-Provided Files
As LLMs process data provided by users, such as documents for summarization or analysis, several compliance challenges arise. Sensitive data in user files must be strictly protected under GDPR, which means systems must identify and handle such data properly.
Users must give informed consent to data processing and have clear options to withdraw their consent at any time. In addition, complying with the right to erasure and the right to be forgotten must be operationally feasible in LLMs so that companies can honor requests from individuals to delete their data from the system.
The good news is that private LLMs can be a viable solution to address privacy concerns and help with GDPR compliance, especially for enterprises handling sensitive or personal data.
Are Private LLMs a Solution?
A private large language model is trained on proprietary data, runs in a controlled environment, and is not exposed to external data inputs that could jeopardize privacy. Here’s how enterprises can benefit from private LLMs:
Control Over Data
A primary advantage of private LLMs over public LLMs is greater control over the data used for training and operations. By using their own data, organizations can implement strict access controls and data handling procedures that comply with GDPR.
Customized Compliance
Private LLMs can be specifically designed to comply with GDPR requirements. Companies can tailor models to use only the essential data needed for specific tasks and directly integrate advanced privacy-enhancing technologies (like differential privacy or federated learning) into their LLMs.
Data Localization
A private large language model can be configured to operate entirely within a specific jurisdiction, such as within the member states of the EU. They can ensure that data does not cross certain borders to comply with strict rules on data transfers outside the EU. In addition, the fact that the data is stored and processed in one jurisdiction simplifies the logistics of responding to requests for access, rectification, or deletion under GDPR.
Reduced Risk of Third-Party Data Exposure
Because private LLMs don’t rely on third-party providers, they eliminate some risks associated with data sharing and processing. Private LLMs allow organizations to directly oversee all aspects of data collection, processing, and model training. They reduce reliance on third-party compliance with GDPR, which can sometimes be a point of vulnerability.
Custom Security Measures
Enterprises can implement customized security protocols tailored to their operational requirements and risk assessments. For example, they can encrypt their data and model interactions more strictly, or access to the LLM can be rigorously regulated to minimize unnecessary disclosure of sensitive data.
Tips for Staying in Line with GDPR
As you know by now, ensuring GDPR compliance when your organization deploys LLMs requires careful planning and robust data management practices. Here’s a structured approach to help your company comply:
1. Understand the Scope of GDPR
Before deploying LLMs, understand which aspects of GDPR apply to your operations, especially regarding data collection, processing, and storage. This includes knowing what constitutes personal data, understanding individuals’ rights concerning their personal information, and identifying your role as either a data processor or data controller.
2. Ensure a Lawful Basis for Data Processing
Identify and document your lawful basis for processing personal data with LLMs. Common elements and examples of lawful bases can include obtaining clear, informed consent from individuals before their data is collected and processed. Another one is proving that processing is necessary for legitimate interests pursued by your business (provided these interests do not override the fundamental rights and freedoms of the people whose data you use).
3. Manage the Rights of Individuals
Ensure mechanisms are in place to address the rights of individuals effectively, including:
- Right to access. Individuals can request access to their personal data processed by the LLM.
- Right to rectification and erasure. Provide means for individuals to have data corrected or erased.
- Right to object. Allow individuals to object to certain uses of their data.
4. Organize Regular Training
Train your staff on GDPR compliance regularly, especially those involved in developing, managing, or operating LLMs. Awareness programs should cover the importance of privacy, data protection strategies, and the legal implications of non-compliance.
5. Document Everything
Keep detailed records of all data collection and processing activities, including the reason for collection, purpose of processing, data categories processed, and details of data transfers. This documentation is crucial for demonstrating compliance, should regulators audit your operation.
Integrating these strategies into your business practices allows you to deploy LLMs that align with GDPR requirements. This protects your company from legal risks and builds trust with your customers and stakeholders.
Conclusion
Integrating LLMs into your business while complying with GDPR is not easy, but it’s most certainly doable. GDPR requires lawful data collection and processing, strong security measures, and respect for individuals’ rights, including obtaining consent in clear and plain language and conducting risk assessments. Therefore, businesses should cultivate robust data management, provide regular compliance training, and keep detailed documentation.
One approach to protecting privacy and improving data control and compliance is to use private LLMs, and this is precisely what we can help you with. Are you ready to incorporate GDPR-compliant generative AI? Contact Dynamiq today to learn about our suite of AI tools. Discover how we can help you create and train private LLMs that safeguard your data with secure processing and mechanisms that honor user rights.